Project details
Funding: MSCA Postdoctoral Fellowships 2022. HORIZON-MSCA-2022-PF-01
Project coordinator: IMDEA Materials
Project period: 01/04/2023 – 31/03/2025
IMDEA Materials' researchers
Supervisor: Dr. Javier LLorca Martínez
Fellow: Valentín Vassilev Galindo
Abstract
Hydrogen energy storage offers a unique combination of scalability, long-term storage, and portability, leading to the so-called hydrogen economy. The major challenge in the hydrogen economy is related to hydrogen production from water and energy generation by hydrogen oxidation into water. In this regard, the main objective of the project High-throughput Discovery of Catalysts for the Hydrogen Economy through Machine Learning (HighHydrogenML) is to develop a high-throughput strategy based on first principles calculations and artificial intelligence tools to discover intermetallic compounds whose catalytic activity can be tuned to reach an optimum catalytic performance for the Hydrogen Evolution Reaction (HER) and Oxygen Reduction Reaction (ORR) using elastic strain engineering.
The successful completion of these objectives will provide unique information for the experimental synthesis of intermetallic compounds with high catalytic activity for the HER and ORR and could, therefore, open a new avenue for a feasible and efficient hydrogen economy. Moreover, the strategies and tools developed in this project can be applied later to many other catalytic processes of large industrial and/or environmental interest. To achieve these goals, the project HighHydrogenML involves multidisciplinary expertise in solid-state physics, materials science, machine learning, and chemistry that will be coupled in a seamless framework to exploit the high predictive power of ab initio calculations in conjunction with the efficiency of ML models. Therefore, this project brings together a researcher with expertise in atomistic and materials modelling within a broad range of different computational chemistry methods and artificial intelligence techniques, a world-recognized supervisor in the area of multiscale modelling of materials, and a research institute with a record of excellence, technology transfer, and top-level training in Materials Science and Engineering.
Partners
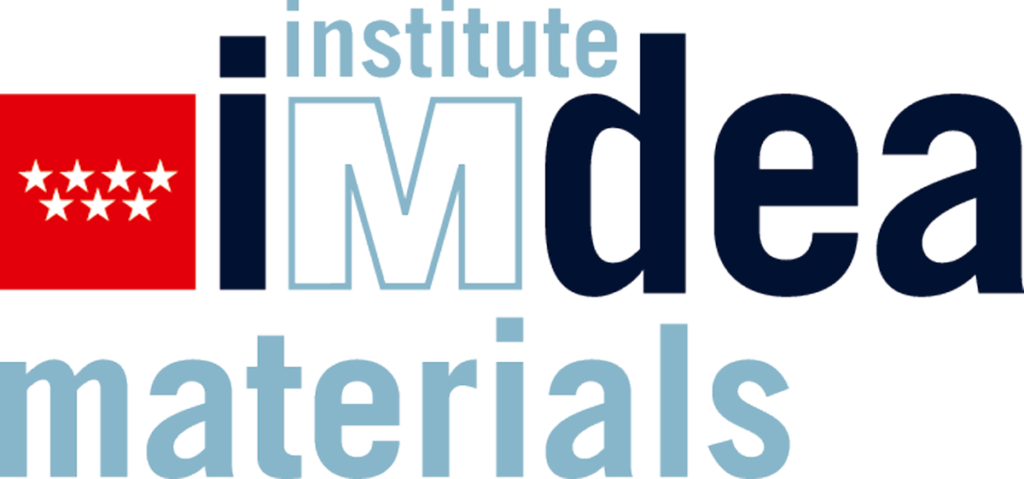
Funded by
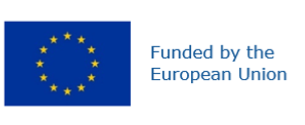
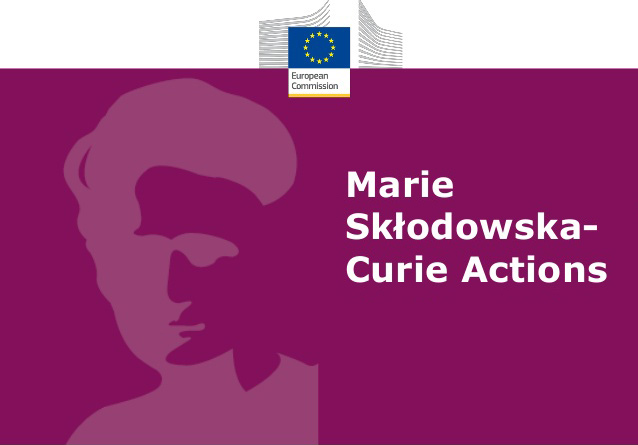
Funded by the European Union under Grant Agreement 101105610. Views and opinions expressed are however those of the author(s) only and do not necessarily reflect those of the European Union. Neither the European Union nor the granting authority can be held responsible for them.