Project details
Funding: Ministerio de Ciencia, Innovación y Universidades – Agencia Estatal de Investigación (AEI)
Project coordinator: Fundación IMDEA Materiales
Project period: 01/09/2024 – 31/08/2027
IMDEA Materials' researchers
Abstract
Polymer nanocomposites are an exciting class of materials with applications in energy, transport and commodity products. Although currently the majority of produced nanocomposites still involved synthetic polymer matricies, this situation can changed by incorporation of emerging bio-based as well as recycled polymer feedstocks. Nevertheless, formulating the composition of a sustainable and multifunctional nanocomposite tuned to a specific application poses a significant challenge. The challenge comes from the fact that the optimal nanocomposite formulations need to include the appropriate components to ensure desired performance while simultaneously addressing constraints regarding the physicochemical compatibility between them and the overall dependence on the processing involved, and which is further complicated by lower techno-mechanical stability of sustainable polymers. These factors make experimentation virtually an indispensable part of the design process, though the endeavor is further complicated by many additional factors, some of which are difficult to control, and manifested by an experimental noise. These circumstances make the design process time consuming and very costly.
The objective of this project is to develop and demonstrate a novel approach to design and optimization of nanocomposite materials, which
would leverage the state-of-the-art machine learning as well as the commoditization and democratization of robotic and automation technologies. Our goal is to increase the throughput of an experimental setup by ca. two orders of magnitude, allowing us to efficiently assess the emerging polymer feedstocks such as bio-based and recycled materials. Furthermore, the increased repeatability of the
experiments involved will give us unprecedented insight in experimental noise, and can be incorporated with our workflow using ML-based
uncertainty quantification approaches.
Finally, the data coming from well-planned experiments, i.e. Bayesian-based Design of Experiments, will ensure proper coverage of the material space, and will be suitable to guide the experimental efforts towards optimal compositions as well as explore further machine learning models. The latter, for example, can help to quickly asses difficult to measure properties e.g. rheological properties of polymer melts, with a set of simple experiments, e.g. by using transfer learning methods. The newly developed capabilities will be used to characterize representative of the emerging bio-based polyamides as well as recycled polypropylene matrixes with representative fillers.
Partners
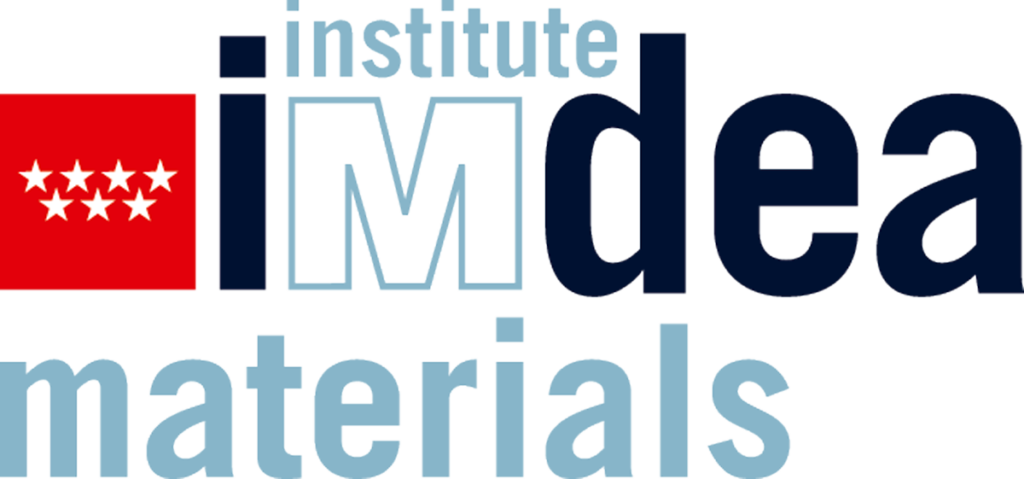
Funded by

Project PID2023-150813OB-I00 , funded by MICIU/AEI/10.13039/501100011033 and FEDER, EU